The trouble with predictions Research Brief | 18th edition – April 2018
"It's tough to make predictions, especially about the future."
Those familiar words are no less true in the world of economic forecasting. A new study considers how far into the future it makes sense to forecast.
Since 2016, Eurosystem staff have been producing macroeconomic projections for the euro area for up to three years into the future. Are forecasts for that kind of horizon still capable of delivering useful insights? The first step in answering that question is to measure a forecast's predictive power. A forecast is typically deemed to be informative if it outperforms what is known as a mean forecast, where the prediction is based only on the average value of the target variable and excludes any other determinants. Moreover, a forecast can only ever be informative if the relationship between the forecast and the target variable is positive, ie if there is a positive correlation.
The further a forecast extends into the future, the greater the typical margin of error and, by extension, the lower its informative value. For this reason, it is crucial to gauge the horizon from which a forecast will no longer be useful. One can gain a rough idea of that horizon by viewing the average dispersion of forecast errors in relation to the average dispersion of errors in the mean forecast over various horizons, which was the method used by Bundesbank economists in the Bundesbank's June 2014 Monthly Report. The results suggest that forecasts of major macroeconomic variables for two years ahead are no longer informative as the average dispersion of forecast errors for this horizon exceeds the average dispersion of errors in the mean forecast. However, this finding is purely descriptive. To determine whether a forecast really is uninformative, it is necessary to carry out statistical tests.
Statistical tests shed light on informative value
In our study, we develop two tests which can be applied to a standard measure of dispersion, namely the mean-squared prediction error. For the mean forecast, this dispersion equals the variance of the target variable. Variance, in turn, is an important measure of dispersion in statistics. The first test directly compares the ratio of the mean-squared prediction error to the variance. However, this test is often only reliable to a degree for small sample sizes, which are the norm in macroeconomic forecasting. The second test explores the correlation between forecasts and target variables and delivers relatively robust results, even in small samples. Both tests are initially applied to the shortest forecast horizon. In macroeconomic forecasting, this is often the current quarter, as the value of the target variable is still unknown at this point in time. If the forecasts for this horizon turn out to be informative, the next-longest horizon, eg the next quarter, is examined. We proceed in this manner until we reach a horizon for which the tests indicate that the forecasts no longer have any predictive power. The horizon before that is then deemed to be the longest for which informative forecasting is still possible. One of the assumptions these tests are based on is that the mean of the target variable does not change over time.
We apply these tests to Consensus Economics forecasts. These forecasts are derived from the average value of various individual forecasts by research institutions and other professional analyses. The accuracy of Consensus forecasts is deemed to be high and often cannot be improved upon by alternative methods as, for instance, Ang et al (2007) found to be the case for inflation forecasting.
We examine forecasts for quarterly real gross domestic product (GDP) and consumer prices (CPI) data up to six quarters ahead. While the GDP forecasts refer to quarter-on-quarter percentage growth rates adjusted for seasonal effects, those for consumer prices relate to the percentage growth rate on the same quarter one year earlier, given that there is often a lack of seasonally adjusted data for consumer prices. These are forecasts produced for the euro area and the G7 countries – a pool of major industrial countries, including the United States, the United Kingdom, Japan and Germany. Forecasts are available from 1995 to 2016 for the G7 countries and from 2002 to 2016 for the euro area.
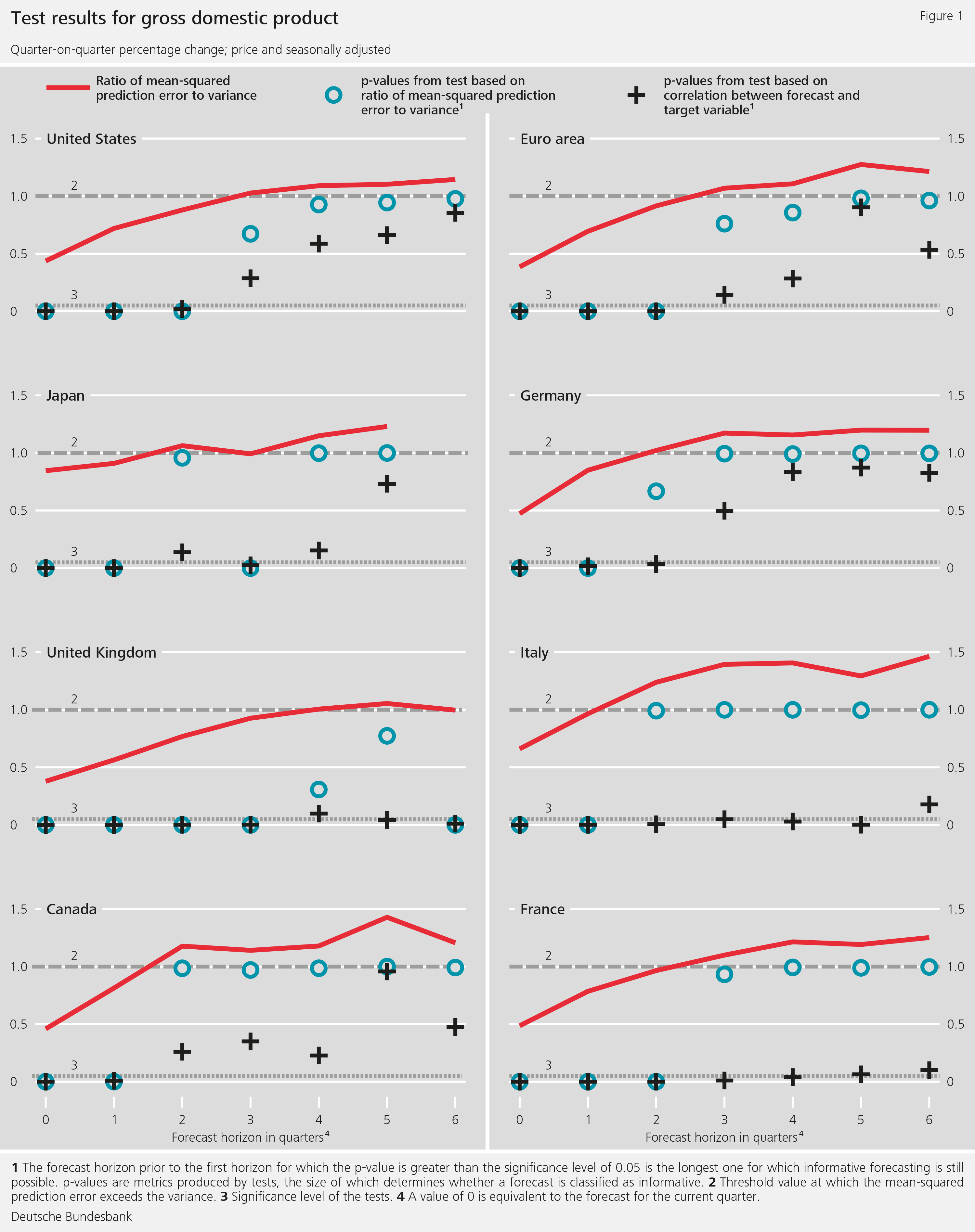
As figures 2 and 3 show, the mean-squared prediction errors for the current and following quarter are usually significantly smaller than the variance of the target variable. Both tests show that this is no coincidence because the forecasts for both these horizons are indeed informative. But in individual cases, things might already look rather different for GDP forecasting in the next quarter but one.
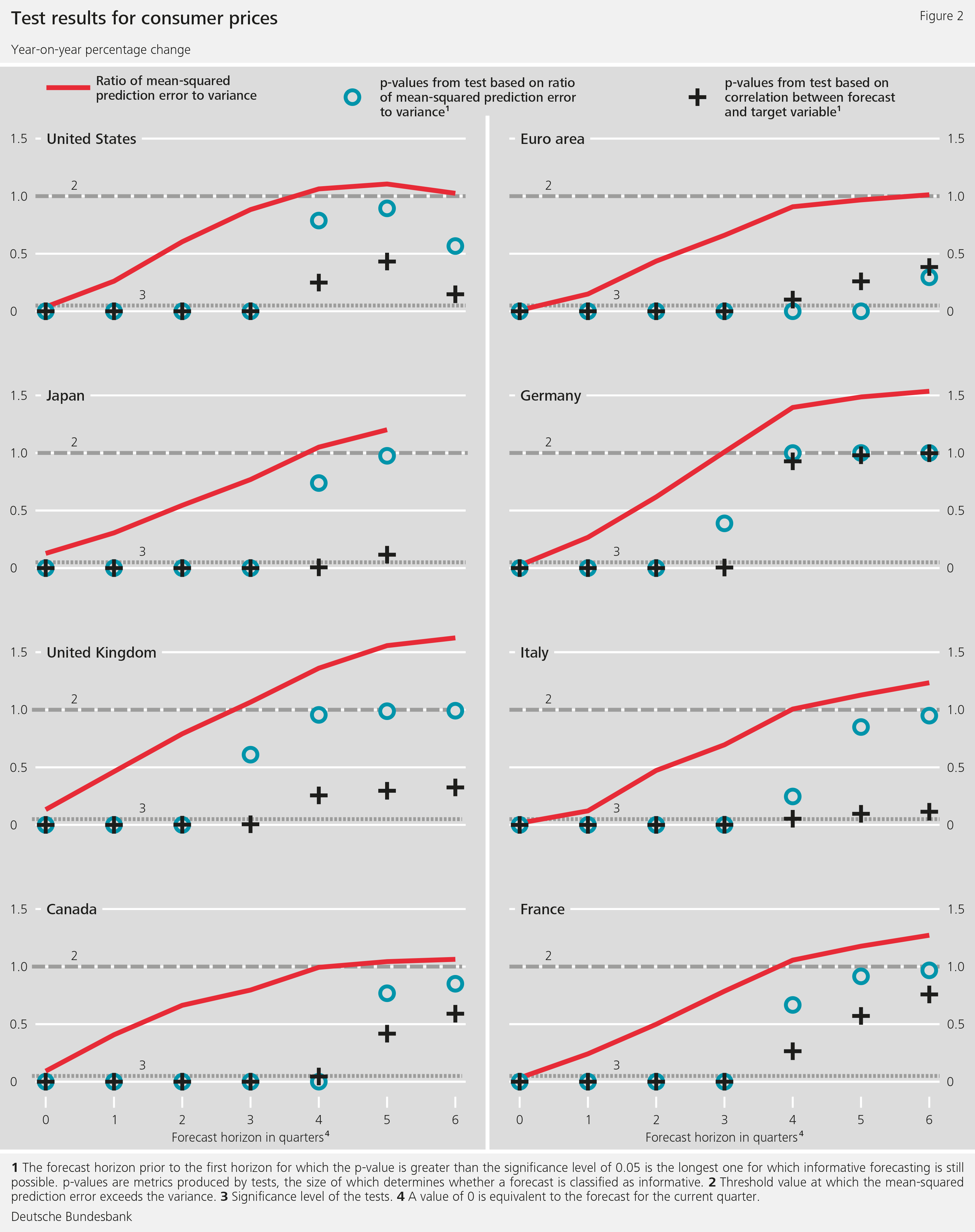
As figure 3 shows, the two tests do not always deliver consistent results. They do, however, both indicate that on average, across countries, GDP forecasts are only informative for two or so quarters ahead. By contrast, CPI forecasts only cease to be informative on average after three quarters.
The role played by the definition of growth rates
Now, does this mean that it is easier to predict CPI growth rates for three quarters ahead than GDP growth rates? Actually, this conclusion would be premature. Differences in the definitions of growth rates impact directly on the forecasts' predictive power. If a year-on-year forecast ceases to be informative after three quarters, this suggests that the forecast for the quarter-on-quarter rate is only informative for the current quarter and already useless for the following quarter. The test results would indicate, then, that it may even be possible, on the whole, to produce informative forecasts for GDP growth rates that reach slightly further into the future than for CPI growth rates.
Examining the quarter-on-quarter rates delivers a more accurate picture of a forecast's actual informative value, because year-on-year rates are also affected by what are known as base effects, which are not factored into the simple mean forecasts. This is because year-on-year rates are roughly equal to the sum of four consecutive quarter-on-quarter rates. If we forecast, say, the year-on-year rate for the first, as yet unknown quarter, then the sum of the three already known quarter-on-quarter rates represents the base effect. This effect has a strong impact on the year-on-year rate that is to be forecasted. This information is not used by the mean forecast, however. It is therefore straightforward to construct a forecast that is informative within the meaning of the definition for year-on-year rates for two quarters ahead by simply using the mean as the forecast for the three unknown quarter-on-quarter rates (the current quarter and the two following ones), while also taking into account the base effect resulting from the preceding quarter.
How accurately can, for example, the inflation rate be predicted three years in advance? Here again, this ultimately depends on how the inflation rate in question is defined. Forecasts are often based on growth rates of average values derived from consecutive calendar years. The inflation rate for 2017, for instance, is the growth rate of the average CPI in 2017 with respect to the average CPI in 2016. This definition likewise has an underlying base effect known as the carry-over effect; this is examined by Tödter (2010), amongst others. Due to the carry-over effect, only the quarter-on-quarter rate for the second quarter of the year after next needs to be forecasted in an informative manner in order to obtain informative forecasts for three calendar years ahead as well. However, since this quarter-on-quarter rate would need to be forecasted at least six quarters ahead, our results imply that it is not possible to produce informative forecasts for three calendar years into the future either for the inflation rate or the GDP growth rate.
Despite what our results say, it can make sense to produce macroeconomic projections for three years ahead, as the Eurosystem does. For one thing, the sample examined in the study might simply be too small to ascertain any informative value for longer forecast horizons. For other, it may well be that the assumptions made in the tests do not hold. This includes the assumption that there is no permanent change in the mean of the target variable over time. Most importantly, however, unlike the Consensus Economics forecasts, the staff projections are based, amongst other things, on certain interest rate assumptions about the future. Over longer horizons, especially, the projections should be regarded less as forecasts and more as scenario analyses which shed light on the interrelationships influencing economic developments.
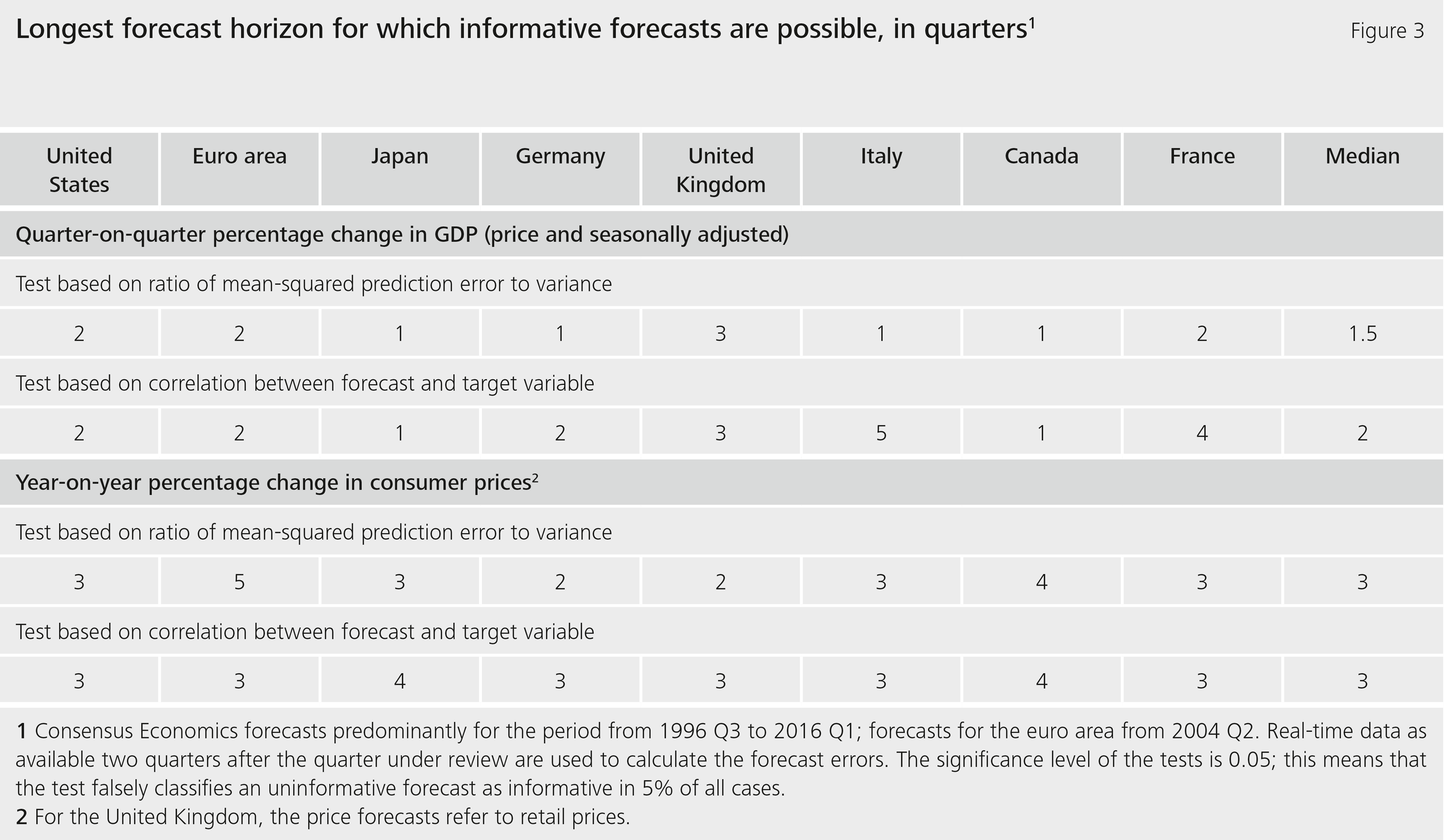
Conclusion
Macroeconomic projections often look far into the future. In our study, we develop new tests to help determine the longest horizon for which forecasting, in the strict sense of the term, is informative from a statistical perspective. For major macroeconomic variables such as the growth rate of real GDP and the CPI inflation rate, forecasts often appear to be informative only for two to three quarters ahead.
Disclaimer |
The views expressed here do not necessarily reflect the opinion of the Deutsche Bundesbank or the Eurosystem. |
Literature
- A Ang, G Bekaert and M Wei, 2007, "Do macro variables, asset markets, or surveys forecast inflation better?", Journal of Monetary Economics, Elsevier, 54(4), pp 1163-1212.
- J Breitung and M Knüppel (2018), "How far can we forecast? Statistical tests of the predictive content", Bundesbank Discussion Paper (07/2018).
- Deutsche Bundesbank (2014), "How informative are the point forecasts in the Bundesbank's macroeconomic projections?", Monthly Report, June 2014, pp 25-28.
- K-H Tödter (2010), "How useful is the carry-over effect for short-term economic forecasting?", Bundesbank Discussion Paper (21), Discussion Paper Series 1: Economic Studies.
The authors | |
![]() Jörg Breitung Professor of Econometrics at the University of Cologne |
![]() Malte KnüppelResearch Economist at the Deutsche Bundesbank’s Research Centre |
News from the Research Centre
Publications
- “Time-varying return correlation, news shocks, and business cycles“ by Norbert Metiu (Deutsche Bundesbank) and Esteban Prieto Fernandez (Deutsche Bundesbank) will be published in the European Economic Review.
- “The Hockey Stick Phillips Curve and the Effective Lower Bound” by Philipp Lieberknecht (Deutsche Bundesbank) and Gregor Boehl (Universität Bonn) will be published in the Journal of Economic Dynamics and Control.
Events
- Spring Conference on Expectations of Households and Firms
24. – 25.04.2025 | Eltville am Rhein
3 MB, PDF